Healthcare Fraud Detection Using Predictive Analytics
Learn about the newest healthcare fraud detection methods based on predictive analytics.
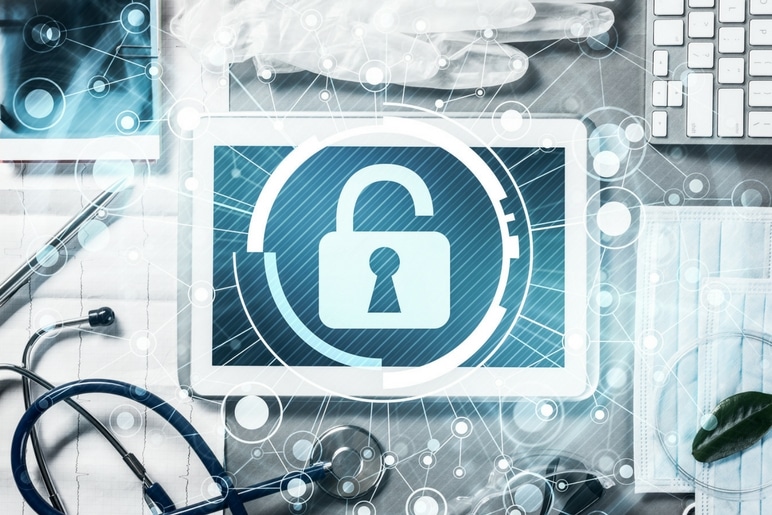
Table of Contents
Those who still watch nightly national news programs are occasionally shown a “raid” on the office or home of a healthcare fraudster. These are people or groups who are usually scamming the government by false claims associated with Medicare and Medicaid. Some of the schemes are quite simple, some quite sophisticated.
Most of the fraud, according to the National Healthcare Anti-Fraud Association, is committed by organized crime groups and a small percentage of healthcare providers. And while we cheer that these scammers are finally caught, the fraud problem is stubborn and is not going away.
Unfortunately, it results in higher costs for patients, cuts into profit margins of payers, and wastes taxpayer dollars that support Medicare and Medicaid.
Table of Contents
Healthcare Fraud: The Numbers
Healthcare is a $2.7 trillion industry in the U.S. alone, and it is estimated that one-third is lost due to different forms of waste, mismanagement, and abuse. Waste includes such activities as providers prescribing unnecessary and redundant testing, devices and medications that are not better than the cheaper ones already in use, etc.
The common examples of fraud and abuse in healthcare include the following:
- Illegal medical billing practices in which claims are falsified.
- Multiple claims are filed by different providers for the same patient.
- Patient identities are stolen and used to gain reimbursement for medical services never provided.
- Collusion between unprincipled providers and their patients in which money from claims is shared.
It is estimated that 3%-10% of annual healthcare costs in the U.S. can be attributed specifically to fraudulent billing.
Further, a recent GAO report states that 68% of all medical fraud is the result of false billing and that healthcare providers are complicit in 62% of those cases, while patients are complicit in 14% of those.
Traditional Methods of Healthcare Fraud Detection
Up until now, efforts to detect healthcare fraud and abuse have involved laborious, feet-on-the-ground investigative work – work that occurs after payments for false claims have been made. And it can take years to gather enough evidence to make arrests and prosecute.
Even if those efforts are successful, there is the issue of recovering the money, and this, too, can take years. It involves legal receiverships and fees, liquidation of the perpetrators’ assets, and with only a percentage recovered in the end.
It’s very much like the old adage of “closing the barn door after the horse has escaped,” rather than taking steps to prevent the horse from escaping in the first place.
The Solution – Healthcare Fraud Prevention With Big Data and Analytics
Clearly, the traditional healthcare fraud detection methods are not working. The more effective way to prevent fraud and abuse is to identify it before claims are paid. And that is why healthcare payers have now embraced the same predictive analytics that other sectors of the economy know to work.
Banks, for example, mine data that links consumer demographics and behaviors to predict the types of loan products that will be the most popular. Life, auto, and home insurance companies mine data to predict risk levels.
Now, it makes sense to approach fraud detection in healthcare using data mining techniques too.
How Predictive Analytics Works to Combat Fraud
Predictive analytics identifies patterns that are potentially fraudulent and then develops sets of “rules” to “flag” certain claims. For example, a provider making a claim for a procedure that is outside of his/her area of expertise would be flagged for further scrutiny, because that is one of the “rules”.
But built into this healthcare fraud detection software model is AI, which will continually mine data, identify more and more emerging fraudulent patterns and create new “rules” for those as well. The “intelligence” in the system learns from these new rules and continually becomes more sophisticated in identifying, even more, fraud potentials. And the best models not only flag the potentials but provide the reasons for that flagging, so that investigations and assessments by management can be completed efficiently.
In short, a solid healthcare fraud auditing and detection system will provide protection to the payer in the following ways:
- Identify inconsistencies and “rule-breaking” behaviors.
- Detect and prevent potentially improper payments, by flagging them for review.
- Continually mine data to identify new fraudulent patterns and develop new “rules” for those as well.
The beauty is in the big data that can all be mined and analyzed by one software tool, rather than a host of separate healthcare fraud detection systems that do not function in coordination, or worse, do not even “know” to check other Internet data sources. One of the most common types of fraud, for example, is the continued claims for an individual who has died. An antiquated system will not have this information, but a system that is “plugged into” big data will.
Several Analytics-Based Approaches For Healthcare Providers
Within a fraud protection services system, there should be built several approaches, and the system itself can use these approaches as determined to be most fitting:
Predictive Modeling in General
This term is the overarching one that is used for all varieties of analytical methods for collecting and analyzing big data to craft a statistical model of behaviors in the future. Analysts will make predictions and then train the model to recognize and engage in monitoring behaviors as claims come in. There is both prepayment modeling, with a focus on such things as office visits and transactions, and post-payment modeling which analyzes both provider and patient behaviors.
Link Analysis
In short, this is a method of analyzing data to detect the relationships among people, providers, and claims. It has been in use by other sectors, public and private, to detect fraud, crime, and even terrorist threats. The auto insurance industry uses it to uncover fraud rings involved in “crash-for-cash” schemes. For fraud protection in healthcare claims, algorithms can evaluate connections between individuals, providers, employees, and even suppliers of equipment, supplies, and pharmaceuticals. False identities can be unearthed, along with fake or multiple addresses and phone numbers. All of these connections can be evaluated to “flag” potential fraud, deny claims, and inform law enforcement.
Duplicate and Gap Testing
To refine the data even further, the system could be trained to conduct duplicate testing to uncover matching transactions for payments, insurance claims made or expenses listed in various reports.
Gap testing, on the contrary, will help the system to identify missing values in sequential data, meaning that you could easily find non-accounted spendings and/or income.
Automated Entry Dates Validation
This technique could be used to identify compromised data. Data entries made during inappropriate times or behindhand can be sent for further investigation and require clarifications.
Risk Scoring
This is a predictive model that can evaluate healthcare providers based on analyzing their claims, billing, and other pertinent data. There can be any number of variables built in, and scores can be attributed based on those variables. Usually, the higher the score the greater the risk, and beyond a certain score, a provider can be flagged for an analyst to dig deeper and look at scores of individual variables. Think of it as similar to a credit score, based on several criteria.
Spike Analysis
This is an approach that will flag obvious changes in the behavior of an individual patient or a healthcare provider. There are normal “spikes” and there are abnormal ones. For example, a primary care physician may see more patients during flu season, and claims will spike. Based upon that provider’s history, such a “spike” will not be seen as abnormal. Spikes that occur at odd times and not within normal parameters for that provider, however, can be flagged for further inquiry. A sudden increase in patients, for example, may indicate that the provider’s identity has been stolen and is being used fraudulently. The system will use stratification of numbers to identify remarkably low or high entries.
Cluster Analysis
This type of predictive modeling puts providers, for example, into clusters, based on predetermined criteria, or parameters. They can then be compared to one another to see patterns that are normal or those that veer from that normality. Analysts can determine custom and unique clusters dependent upon payers’ needs, and use those needs as a guide to create the right algorithms.
Clustering is just one example of unsupervised anomaly detection methods – “smart” models, which would analyze all the data at disposal and automatically mark data sets, which do not seem to correspond the general pattern.
More precise results could be achieved by using DBSCAN (Density-based spatial clustering of applications with noise) or Hidden Markov Model, which allows to base predictions on larger chunks of past data aggregated within your system.
Trend Analysis
The population is growing older. Predictive analysis can be used to devise models related to the medical needs of the future, as people live longer. It can also be used to predict that preventive care which may best keep an aging population healthier. In terms of fraud prevention, it can also prove valuable. If a provider, for example, is providing healthcare services which fall outside the parameters of a trend, let’s say the percentage of aging patients who require a certain procedure, that can be fagged for assessment.
The Benefits Of Predictive Analytics are Pretty Obvious
Like in any other insurance sector, fraud is expensive. For decades, payers have attempted to figure out how to reduce fraud. Predictive analytics is now providing the tools to do just that, with technology that does all of the heavy lifting and provides a number of benefits.
Cost Savings and Protection
Identifying fraud up front means no more chasing the criminals after payments have been made, along with the investigative and legal costs. It’s just a much smarter way to do business. And whether that cost savings impact your own business bottom line or that of a public entity with whom you are contracted, the results can only be good ones.
Continued Increase in Fraud Prevention Through Continued Learning
As the system continues to “learn,” additional potential fraud will be identified for assessment. It reacts to changes in behavior patterns; it can build provider profiles and perform comparisons; it can establish new “rules” based upon big data.
Payers Can Maintain Compliance with Government Prompt Payment Regs
The amount of manual “chasing” down of claims, whether pre- or post-payment, is significantly reduced. Analysis can happen in real-time, as claims are presented and resolutions achieved much faster.
Whether you are a steward of public dollars or a steward of your own business’s financial health, a predictive analytics software system just makes good sense. If you are ready to talk about your specific needs, let the healthcare analytics experts at Romexsoft explain what we can do for you.